In an increasingly volatile world, businesses are faced with numerous risks ranging from cyber threats to global pandemics. Traditional risk management techniques, while effective in the past, are now being complemented with Resilience-Based Risk Management (RBRM), a more proactive and adaptive approach. By integrating Artificial Intelligence (AI) and Machine Learning (ML), organizations can now strengthen their resilience and ensure quicker recovery in the face of adversity.
1. What is Resilience-Based Risk Management (RBRM)?
RBRM is the process of preparing organizations to not only anticipate risks but also respond effectively and recover quickly from disruptions. It differs from traditional risk management by focusing on adaptability, quick recovery, business continuity, and proactive planning.
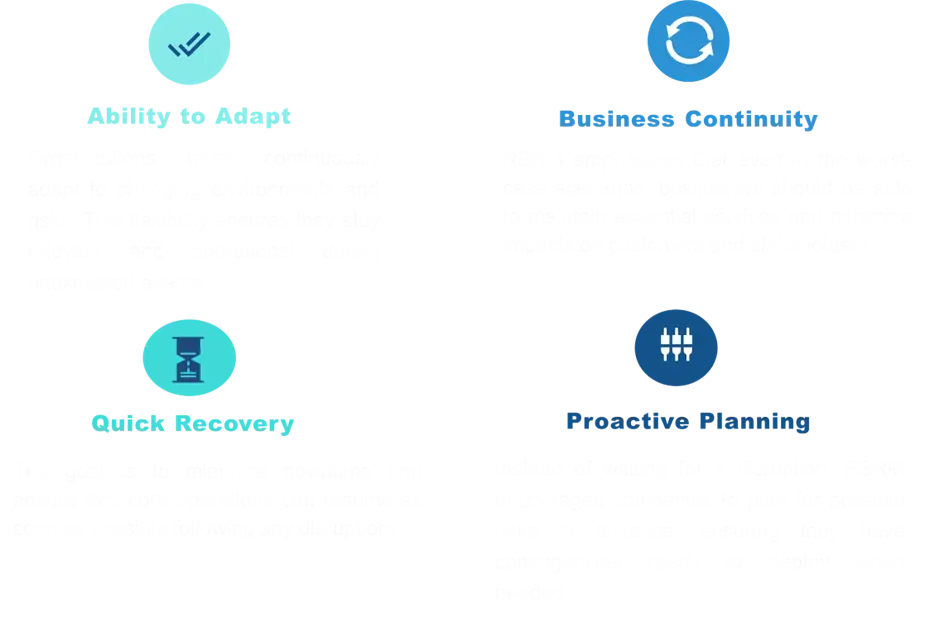
2. Key Elements of Resilience-Based Risk Management (RBRM)
A strong Resilience-Based Risk Management (RBRM) framework involves several critical components that allow organizations to anticipate, assess, and respond effectively to risks. Let’s delve deeper into the three fundamental elements: Risk Assessment, Business Impact Analysis (BIA), and Ongoing Monitoring.
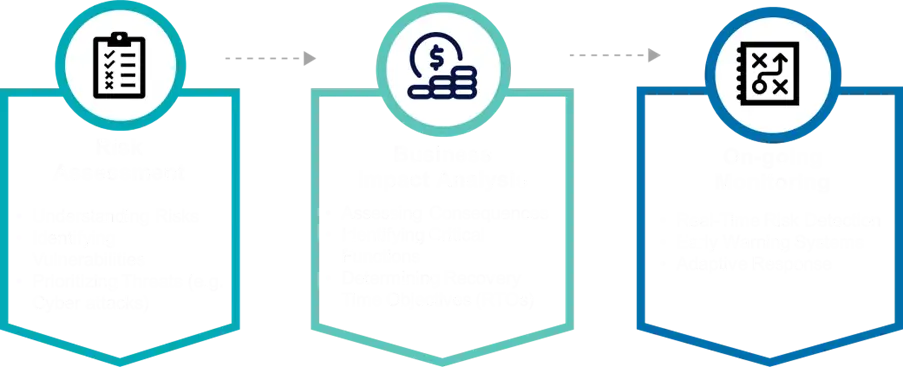
1. Risk Assessment
Risk assessment forms the foundation of any robust risk management strategy. It is the process of understanding potential risks that could affect an organizationโs operations, identifying vulnerabilities, and prioritizing threats.
- Understanding Risks: Identify a wide range of internal and external risks, including cyber-attacks, operational inefficiencies, and human errors, using AI to analyze historical data and emerging threats.
- Identifying Vulnerabilities & Prioritizing Threats: AI tools help uncover weak points in IT infrastructure, processes, and personnel, and prioritize high-risk threats like cyber-attacks based on likelihood and impact.
2. Business Impact Analysis (BIA)
A Business Impact Analysis (BIA) is a key element of RBRM that focuses on understanding how disruptions would affect an organization. It evaluates the consequences of potential disruptions and helps in defining recovery strategies.
- Assessing Consequences & Identifying Critical Functions: AI-driven simulations model disruptions (e.g., natural disasters, IT failures) and highlight vital business functions like customer service and supply chains that need protection.
- Determining Recovery Time Objectives (RTOs): AI helps set optimal RTOs to restore critical operations, ensuring minimal downtime and damage in case of incidents.
3. Ongoing Monitoring
Ongoing monitoring is a proactive process that ensures that potential risks are continuously detected, analyzed, and mitigated in real-time. This is particularly crucial for staying ahead of dynamic threats like cybersecurity issues or natural disasters.
- Real-Time Risk Detection & Early Warning Systems: AI-powered tools analyze real-time data, detect emerging threats (e.g., fraud, cybersecurity), and provide early warnings to avert risks.
- Adaptive Response: AI systems automatically trigger preemptive actions, such as isolating compromised systems or notifying personnel, reducing response time and improving resilience.
3. Role of AI and Machine Learning in RBRM
AI and Machine Learning (ML) play a critical role in modernizing and enhancing the traditional approach to Resilience-Based Risk Management (RBRM). These technologies enable organizations to analyze vast amounts of data, predict risks with greater accuracy, and automate decision-making processes, ensuring a more resilient and proactive risk management framework. Letโs explore how AI and ML contribute to the key pillars of Data Analysis, Prediction, and Automation.
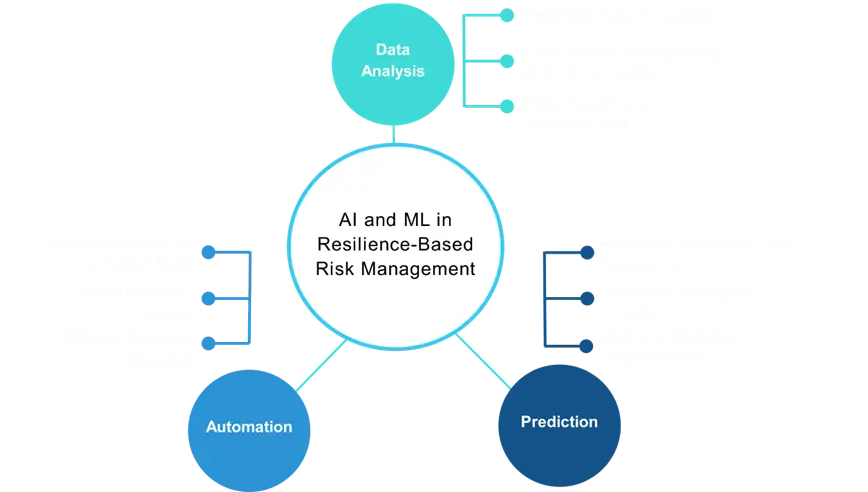
Data Analysis:
- Real-time Data Processing: AI analyzes vast data instantly, enabling real-time risk detection.
- Unstructured Data Handling: Processes data from unconventional sources like social media for early warning signs.
- Deep Insights into Historical Data: ML identifies hidden patterns in historical data to anticipate future risks.
Prediction:
- Advanced Analytics for Risk Forecasting: AI forecasts risks with precision, enhancing preparedness.
- Identifying Emerging Threats: Detects anomalies and early signals of potential crises.
- Scenario Modeling for Preparedness: Simulates various risk scenarios to improve response strategies.
Automation:
- Rapid Response via Automated Alerts: AI-triggered alerts ensure fast mitigation actions.
- Smart Decision-Making: Provides real-time, data-driven insights for informed decisions.
Efficient Resource Allocation: AI optimizes resource deployment to minimize crisis impacts.
5. Top Industries Benefiting from RBRM
Several industries are already leveraging RBRM with AI and ML to enhance their risk resilience.
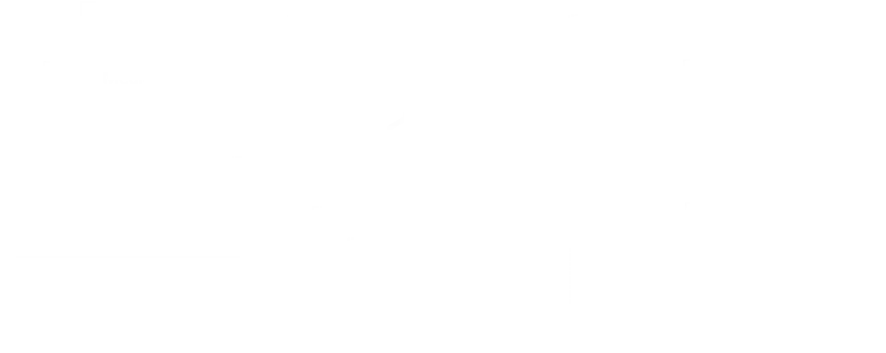
Case Study: RBRM in Fraud Detection and Cyber Risk Management for a Bank
With the rise in sophisticated cyber attacks and digital fraud, a leading retail bank faced increasing vulnerabilities to fraudulent activities, including phishing scams, account takeovers, and unauthorized transactions. Traditional cybersecurity methods were slow in identifying and mitigating threats, leading the bank to adopt Resilience-Based Risk Management (RBRM) powered by Artificial Intelligence (AI) and Machine Learning (ML).
Challenge:
The bank struggled with detecting emerging cyber risks and fraudulent behavior in real time, often discovering issues after substantial financial and reputational damage. This included:
- Cyber Attacks: Increasing attempts to breach customer accounts through phishing, malware, and ransomware attacks.
- Fraudulent Transactions: Unauthorized transactions and account takeovers, which impacted customer trust and regulatory compliance.
RBRM Solution:
The bank implemented an AI-driven RBRM system to enhance its fraud detection and cyber risk management capabilities:
- Fraud Detection: AI algorithms continuously monitored customer transactions, using pattern recognition to detect anomalies such as unusual spending behaviors, location-based discrepancies, and abnormal transaction volumes.
- Cyber Threat Monitoring: Machine Learning models analyzed network traffic and user behavior to detect signs of cyber threats in real time, such as phishing attempts and malware installations.
- Ongoing Monitoring & Early Warnings: AI-based systems provided real-time alerts for both fraudulent activities and cyber risks, enabling the bank to proactively take action before significant damage occurred.
Results:
- Real-Time Fraud Detection & Prevention: AI identified suspicious transactions instantly, flagging them for review or automatically freezing accounts to prevent further fraudulent activity. The system reduced fraud losses by 30% within the first year.
- Cyber Attack Mitigation: The AI system successfully detected malware and phishing attacks early, stopping potential breaches before they escalated. This reduced the risk of data breaches and enhanced customer trust.
- Automated Response & Recovery: In case of detected threats, AI systems automatically triggered security protocols such as multi-factor authentication, account verification, and system isolation, ensuring faster response times and minimal operational disruption.
This case demonstrates how AI-driven RBRM helped the bank achieve a more resilient stance against cyber fraud and attacks, strengthening both its security infrastructure and customer confidence
6. Challenges in AI-Driven RBRM
While AI and ML bring tremendous advantages to RBRM, there are challenges organizations must address:
- Data Privacy and Security AI systems rely on vast amounts of data, making privacy concerns and data security paramount.
- Bias in AI Models AI algorithms can develop biases based on the data they are trained on, leading to inaccurate or unfair predictions.
- Integration with Legacy Systems Many organizations face difficulties integrating advanced AI-driven tools with their existing legacy systems.
- High Implementation Costs Although AI offers long-term benefits, the initial investment can be high for small and mid-sized companies.
Resilience-Based Risk Management, powered by AI and Machine Learning, is quickly becoming the gold standard for organizations looking to thrive in an uncertain world. By adopting this approach, businesses can enhance their ability to adapt, recover quickly, and ensure continuity even during the most disruptive events. With ongoing advancements in AI, the future of RBRM promises even greater agility and foresight, allowing companies to stay one step ahead of risks.