As artificial intelligence (AI) and machine learning (ML) become integral to business operations, organizations face a growing imperative to ensure their models are not only effective but also ethical, transparent, and compliant. Model governance—the systematic oversight of AI models throughout their lifecycle—has emerged as a cornerstone of responsible AI adoption. When seamlessly integrated into the MLOps (Machine Learning Operations) pipeline, model governance ensures that AI systems are developed, deployed, and monitored with accountability, fairness, and trustworthiness at their core.
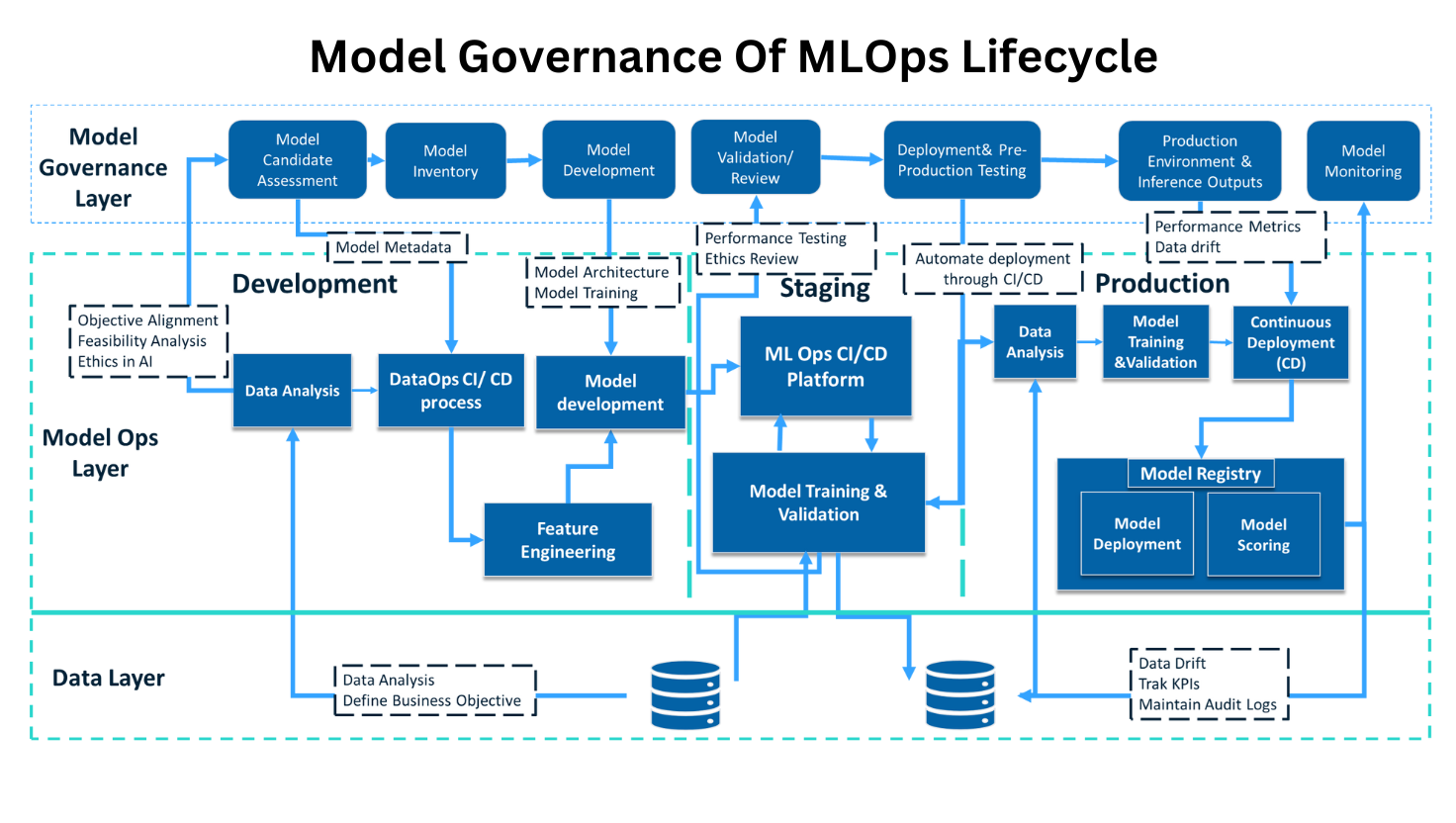
The Strategic Imperative for Model Governance in MLOps
AI models, while powerful, are not immune to risks such as bias, data drift, and unintended consequences. Without proper oversight, these risks can lead to reputational damage, regulatory penalties, and loss of public trust. Model governance addresses these challenges by embedding ethical principles and risk management practices into every stage of the ML lifecycle.
At its heart, model governance is about fostering trust. It ensures that AI systems:
- Are aligned with business objectives.
- Operate transparently and accountably.
- Mitigate risks such as bias, privacy violations, and performance degradation.
- Comply with evolving regulations like GDPR, CCPA, and industry-specific standards.
By prioritizing governance, organizations can unlock the full potential of AI while safeguarding against its pitfalls.
Key Stages of Model Governance in the MLOps Lifecycle
Model governance is not a one-time activity but an ongoing process that spans the entire ML lifecycle. Below, we break down the key stages and highlight best practices for each.
1. Responsible Model Candidate Assessment
Objective: Evaluate the feasibility, ethical implications, and alignment of proposed AI models with business goals.
Before building an AI model, organizations must ask critical questions:
- Does this model address a clear business need?
- Is the required data available, and is it ethically sourced?
- What are the potential risks, such as bias or regulatory non-compliance?
This stage lays the foundation for ethical AI development by ensuring that only models with clear value and minimal risk move forward.
- Objective Alignment: Clearly define business outcomes.
- Feasibility Analysis: Assess data availability and technological readiness.
- Ethics in AI: Ensure fairness, privacy, and responsible data usage.
- Risk Mitigation: Identify potential biases and regulatory challenges.
2. Model Inventory and Metadata Management
Objective: Maintain a comprehensive and organized record of all AI models.
An organized inventory is essential for traceability and accountability. Each model should have:
- A unique identifier (e.g., Model ID).
- Detailed metadata, including data sources, purpose, and ethical considerations.
- Version control to track changes and iterations.
3. Model Development
Objective: Build AI models responsibly, with a focus on fairness, transparency, and ethical data usage.
During development, organizations must:
- Ensure data privacy and informed consent.
- Train models using diverse and representative datasets to minimize bias.
- Document the model architecture, training process, and decision-making logic.
Feature engineering—a critical aspect of generative AI—requires special attention to avoid unintended outputs. Transparency in development fosters trust and makes it easier to audit models later.
4. Model Validation and Review
Objective: Validate models for accuracy, fairness, and compliance before deployment.
This stage involves rigorous testing to ensure models meet performance benchmarks and ethical standards. Key activities include:
- Performance testing to evaluate robustness and reliability.
- Bias audits to identify and mitigate discriminatory outcomes.
- Ethics reviews to ensure alignment with organizational values and regulatory requirements.
Validation is a gatekeeping function that prevents flawed or unethical models from entering production.
5. Deployment and Pre-Production Testing
Objective: Prepare models for real-world use while maintaining ethical safeguards.
Deployment is a critical transition point where models move from controlled environments to live systems. Best practices include:
- Automating deployment through CI/CD pipelines to reduce errors.
- Conducting user acceptance testing (UAT) to validate functionality.
- Implementing ethical AI monitoring to detect unintended behaviors early.
This stage ensures that models are ready to deliver value without compromising ethical standards.
6. Production Environment and Inference Outputs
Objective: Monitor models in real-time to ensure they perform as expected.
Once deployed, models must be continuously monitored for:
- Performance metrics such as accuracy and latency.
- Data drift, which occurs when the input data distribution shifts over time.
- Ethical concerns, such as biased or harmful outputs.
7. Model Monitoring
Objective: Ensure ongoing effectiveness and compliance.
Continuous evaluation is essential to maintain model integrity. Organizations should:
- Track key performance indicators (KPIs) and ethical benchmarks.
- Maintain audit logs for accountability and transparency.
- Address data drift and other anomalies promptly.
Monitoring is not just about performance—it’s about ensuring models remain aligned with ethical and regulatory standards over time.
8. Model Retirement and Attestation
Objective: Decommission models responsibly when they outlive their usefulness.
Models that no longer meet business needs or ethical standards should be retired with care. This involves:
- Evaluating the social and business impact of retirement.
- Conducting post-retirement analysis to determine if reinstatement is feasible.
- Ensuring compliance during the decommissioning process.
Key Pillars of Trustworthy AI
Ethical considerations are at the heart of model governance. To build trustworthy AI systems, organizations must:
- Define Accountability: Clearly assign roles and responsibilities for AI model stakeholders.
- Promote Transparency: Maintain detailed documentation and audit trails.
- Foster Inclusivity: Ensure models are fair and inclusive, avoiding biases that harm underrepresented groups.
- Enable Human Oversight: Retain human intervention in AI-driven decisions, especially in high-stakes scenarios.
By embedding these principles into their governance frameworks, organizations can create AI systems that benefit both businesses and society.
Future Outlook MLOps Governance
As AI continues to evolve, so too will the challenges and opportunities associated with model governance. Emerging trends include:
- Explainable AI (XAI): Techniques to make AI decisions more interpretable and transparent.
- Regulatory Evolution: Stricter regulations around AI ethics and data privacy.
- Automated Governance Tools: Platforms that streamline governance processes and enhance scalability.
Organizations must stay ahead of these trends by investing in advanced governance frameworks that balance innovation with responsibility.
Partner with Asteriqx Consulting for AI Excellence
At Asteriqx Consulting, we empower organizations to harness the power of AI responsibly. With expertise in model governance, MLOps integration, and ethical AI solutions, we are your trusted partner for navigating the future of AI.
Contact us today to learn how we can help your organization stay ahead in the AI revolution.
6 responses to “MLOps Governance : A Practical Guide”
Outstanding insights! The community around Chrome Dinosaur grows stronger each day.
Masterful content! Have you achieved the ultimate creation in AI KungFu? Keep creating!
This resonates deeply with my artistic journey! By the way, Sprunki has been my go-to platform for experimental music creation.
Unleash musical creativity through Sprunki InCrediBox‘s innovative character-based sound design system.
Outstanding post! Sprunki Games shares your creative vision.
Constantly amazed by Hailuo AI KungFu capabilities. Hailuo AI KungFu brings such professionalism.